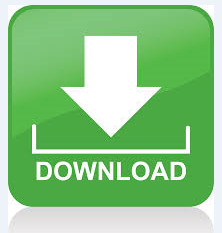
The summary should be informative enough for the reader to get a full understanding of the source paper.
The summary should explain the study aim, protocol, subject population, outcome, and impact on patient treatment and future research. Complex medical concepts should be explained ‘in-context’ with a short plain language definition. Specialist medical terms should be replaced with common language. Summaries should be approximately 250 words. We targeted a complete summary, including important details from the source text like patient population, treatment outcomes, and how the research impacted disease treatment. HypothesisĪ model like OpenAI’s Davinci-3, the original LLM that underpinned ChatGPT, could produce a passable Plain Language Summary of medical text describing a drug-study, which could then be refined by an author or editor in short time. Time for researchers and editors to focus on publishing new research. Our customer requested that we prototype using GPT to produce Plain Language Summaries, freeing up BackgroundĮvery day, hundreds of new medical specialist papers are published on sites such as PubMed.įor patients or caregivers with a keen interest in new research impacting their condition, it can often be difficult to comprehend the complex jargon and language.Ĭonsequently, many journals require submitters to produce a separate short Plain Language Summary for the non-specialist reader.
On a recent engagement, our team created a demo of how to use the Azure OpenAI service to leverage LLM capabilities in generating summaries of medical documents for non-specialist readers. The recent explosion in the popularity of Large Language Models (LLM) such as ChatGPT has opened the floodgates to an enormous and ever-growing list of possible new applications in numerous fields.
In this post we’ll demonstrate some prompt engineering techniques to create summaries of medical research publications. June 27th, 2023 0 1 Large Language Model Prompt Engineering for Complex Medical Research Summarization